The thermal noise of a resistor is an example of a random process in which the value of a variable at any given time is unpredictable, and it is only one of many random processes that generate noise in electronic circuits. We will see that such processes can be described in terms of their statistical measures—that is, in terms of certain. A stochastic process may involve several related random variables. Common examples include the growth of a bacterial population, an electrical current fluctuating due to thermal noise, or the movement of a gas molecule. Stochastic processes are widely used as mathematical models of systems and phenomena that appear to vary in a random manner.
10.1.5 Gaussian Random Processes
Here, we will briefly introduce normal (Gaussian) random processes. We will discuss some examples of Gaussian processes in more detail later on.Many important practical random processes are subclasses of normal random processes.
First, let us remember a few facts about Gaussian random vectors. As we saw before, random variables $X_1$, $X_2$,..., $X_n$ are said to be jointly normal if, for all $a_1$,$a_2$,..., $a_n$ $in mathbb{R}$, the random variable
begin{align}%label{} a_1X_1+a_2X_2+...+a_nX_nend{align}is a normal random variable. Also, a random vectorbegin{equation}nonumber textbf{X} = begin{bmatrix} X_1 %[5pt] X_2 %[5pt] . [-10pt] . [-10pt] . [5pt] X_nend{bmatrix}end{equation}is said to be normal or Gaussian if the random variables $X_1$, $X_2$,..., $X_n$ are jointly normal. An important property of jointly normal random variables is that their joint PDF is completely determined by their mean and covariance matrices. More specifically, for a normal random vector X with mean $mathbf{m}$ and covariance matrix C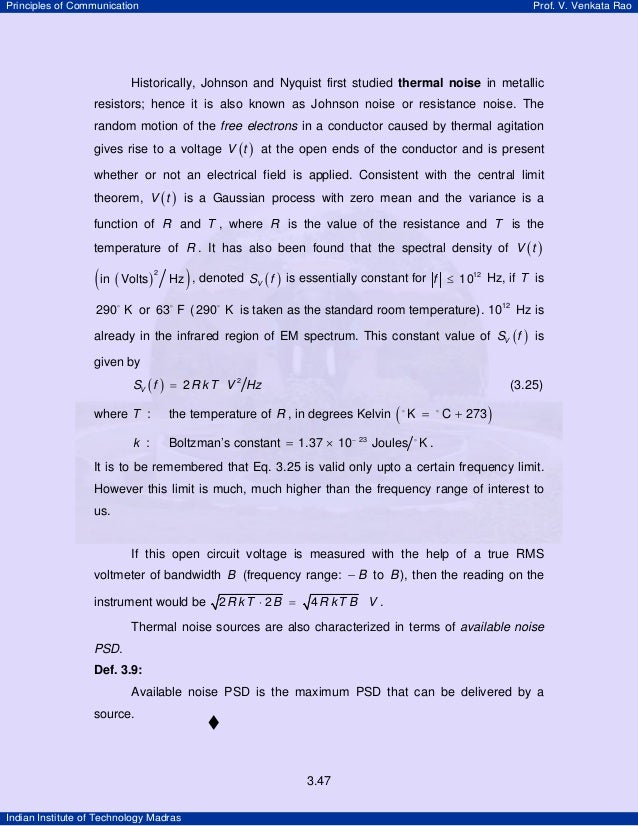
Example
Let $X(t)$ be a zero-mean WSS Gaussian process with $R_X(tau)=e^{-tau^2}$, for all $tau in mathbb{R}$.
- Find $Pbig(X(1) lt 1big)$.
- Find $Pbig(X(1)+X(2) lt 1big)$.
- Solution
- $X(1)$ is a normal random variable with mean $E[X(1)]=0$ and variance begin{align*}%label{} textrm{Var}big(X(1)big)&=E[X(1)^2] &=R_X(0)=1. end{align*} Thus, begin{align*}%label{} Pbig(X(1) lt 1big)&=Phi left(frac{1-0}{1} right) &=Phi(1) approx 0.84 end{align*}
- Let $Y=X(1)+X(2)$. Then, $Y$ is a normal random variable. We have begin{align*}%label{} EY &=E[X(1)]+E[X(2)] &=0; end{align*} begin{align*}%label{} textrm{Var}(Y) &=textrm{Var}big(X(1)big)+textrm{Var}big(X(2)big)+2 textrm{Cov}big(X(1),X(2)big). end{align*} Note that begin{align*}%label{} textrm{Var}big(X(1)big)&=E[X(1)^2]-E[X(1)]^2 &=R_X(0)- mu_X^2 &=1-0=1=textrm{Var}big(X(2)big); end{align*} begin{align*}%label{} textrm{Cov}big(X(1),X(2)big)&=E[X(1)X(2)]-E[X(1)]E[X(2)] &=R_X(-1)-mu_X^2 &=e^{-1} -0=frac{1}{e}. end{align*} Therefore, begin{align*}%label{} textrm{Var}(Y) &=2+frac{2}{e}. end{align*} We conclude $Y sim N(0,2+frac{2}{e})$. Thus, begin{align*}%label{} Pbig(Y lt 1big)&=Phi left(frac{1-0}{sqrt{2+frac{2}{e}}} right) &=Phi(0.6046) approx 0.73 end{align*}
An important property of normal random processes is that wide-sense stationarity and strict-sense stationarity are equivalent for these processes. More specifically, we can state the following theorem.
White Noise Process

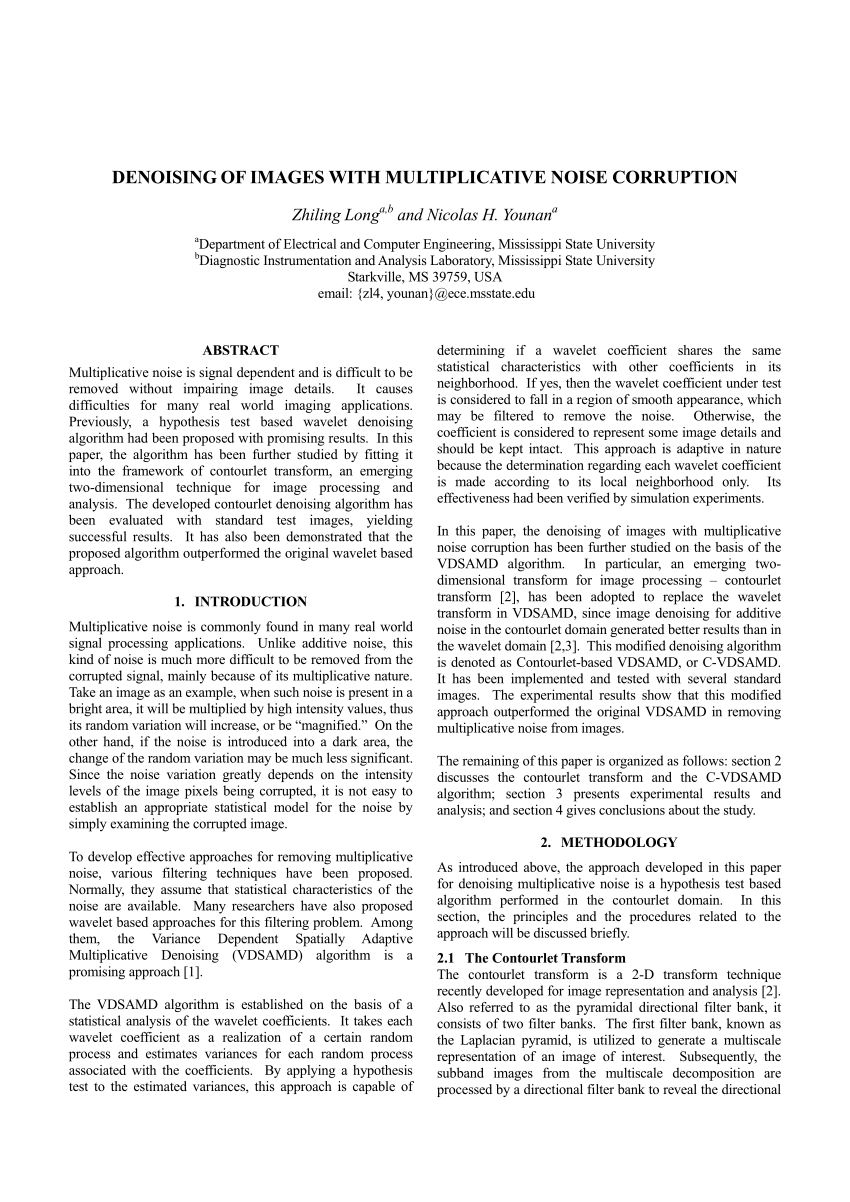
The thermal noise of a resistor is an example of a random process in which the value of a variable at any given time is unpredictable, and it is only one of many random processes that generate noise in electronic circuits. We will see that such processes can be described in terms of their statistical measures—that is, in terms of certain. A stochastic process may involve several related random variables. Common examples include the growth of a bacterial population, an electrical current fluctuating due to thermal noise, or the movement of a gas molecule. Stochastic processes are widely used as mathematical models of systems and phenomena that appear to vary in a random manner.
10.1.5 Gaussian Random Processes
Here, we will briefly introduce normal (Gaussian) random processes. We will discuss some examples of Gaussian processes in more detail later on.Many important practical random processes are subclasses of normal random processes.
First, let us remember a few facts about Gaussian random vectors. As we saw before, random variables $X_1$, $X_2$,..., $X_n$ are said to be jointly normal if, for all $a_1$,$a_2$,..., $a_n$ $in mathbb{R}$, the random variable
begin{align}%label{} a_1X_1+a_2X_2+...+a_nX_nend{align}is a normal random variable. Also, a random vectorbegin{equation}nonumber textbf{X} = begin{bmatrix} X_1 %[5pt] X_2 %[5pt] . [-10pt] . [-10pt] . [5pt] X_nend{bmatrix}end{equation}is said to be normal or Gaussian if the random variables $X_1$, $X_2$,..., $X_n$ are jointly normal. An important property of jointly normal random variables is that their joint PDF is completely determined by their mean and covariance matrices. More specifically, for a normal random vector X with mean $mathbf{m}$ and covariance matrix C, the PDF is given bybegin{align*} f_{mathbf{X}}(mathbf{x})=frac{1}{(2pi)^{frac{n}{2}} sqrt{dettextbf{C}}} exp left{-frac{1}{2} (textbf{x}-textbf{m})^T mathbf{C}^{-1}(textbf{x}-textbf{m}) right}.end{align*}Now, let us define Gaussian random processes.Example
Let $X(t)$ be a zero-mean WSS Gaussian process with $R_X(tau)=e^{-tau^2}$, for all $tau in mathbb{R}$.
- Find $Pbig(X(1) lt 1big)$.
- Find $Pbig(X(1)+X(2) lt 1big)$.
- Solution
- $X(1)$ is a normal random variable with mean $E[X(1)]=0$ and variance begin{align*}%label{} textrm{Var}big(X(1)big)&=E[X(1)^2] &=R_X(0)=1. end{align*} Thus, begin{align*}%label{} Pbig(X(1) lt 1big)&=Phi left(frac{1-0}{1} right) &=Phi(1) approx 0.84 end{align*}
- Let $Y=X(1)+X(2)$. Then, $Y$ is a normal random variable. We have begin{align*}%label{} EY &=E[X(1)]+E[X(2)] &=0; end{align*} begin{align*}%label{} textrm{Var}(Y) &=textrm{Var}big(X(1)big)+textrm{Var}big(X(2)big)+2 textrm{Cov}big(X(1),X(2)big). end{align*} Note that begin{align*}%label{} textrm{Var}big(X(1)big)&=E[X(1)^2]-E[X(1)]^2 &=R_X(0)- mu_X^2 &=1-0=1=textrm{Var}big(X(2)big); end{align*} begin{align*}%label{} textrm{Cov}big(X(1),X(2)big)&=E[X(1)X(2)]-E[X(1)]E[X(2)] &=R_X(-1)-mu_X^2 &=e^{-1} -0=frac{1}{e}. end{align*} Therefore, begin{align*}%label{} textrm{Var}(Y) &=2+frac{2}{e}. end{align*} We conclude $Y sim N(0,2+frac{2}{e})$. Thus, begin{align*}%label{} Pbig(Y lt 1big)&=Phi left(frac{1-0}{sqrt{2+frac{2}{e}}} right) &=Phi(0.6046) approx 0.73 end{align*}
An important property of normal random processes is that wide-sense stationarity and strict-sense stationarity are equivalent for these processes. More specifically, we can state the following theorem.
White Noise Process
Theorem Consider the Gaussian random processes $big{X(t), t in mathbb{R}big}$. If $X(t)$ is WSS, then $X(t)$ is a stationary process.
- Proof
- We need to show that, for all $t_1,t_2,cdots, t_r in mathbb{R}$ and all $Delta in mathbb{R}$, the joint CDF of begin{align}%label{} X(t_1), X(t_2), cdots, X(t_r)end{align} is the same as the joint CDF of begin{align}%label{} X(t_1+Delta), X(t_2+Delta), cdots, X(t_r+Delta).end{align}Since these random variables are jointly Gaussian, it suffices to show that the mean vectors and the covariance matrices are the same. To see this, note that $X(t)$ is a WSS process, sobegin{align}%label{} mu_X(t_i)=mu_X(t_j)=mu_X, quad textrm{for all }i,j,end{align} andbegin{align}%label{} C_X(t_i+Delta,t_j+Delta)=C_X(t_i,t_j)=C_X(t_i-t_j), quad textrm{for all }i,j.end{align}From the above, we conclude that the mean vector and the covariance matrix ofbegin{align}%label{} X(t_1), X(t_2), cdots, X(t_r)end{align} is the same as the mean vector and the covariance matrix ofbegin{align}%label{} X(t_1+Delta), X(t_2+Delta), cdots, X(t_r+Delta).end{align}
Similarly, we can define jointly Gaussian random processes.